발간물
연구보고서
목록으로
미래환율의 변동을 정확하게 예측하는 것은 중앙은행, 금융시장 참가자 및 정책당국자에게 대단히 중요하다. 하지만 현존하는 다양한 경제모형들의 환율 예측력이 크지 않다는 것은 잘 알려진 사실이다. 예를 들어 Meese and Rogoff (1983)가 확률보행 모형이 경제 기초여건(economic fundamentals)의 변동을 반영한 모형보다 오히려 표본 외 샘플에서 환율 예측력이 더 우수하다는 결과를 보고한 이래로 후속연구들은 이러한 결과를 뒤집을 수 있는 강건하고 체계적인 실증분석 결과를 제시하지 못하고 있다.
이에 본 연구는 기존 정형화된 환율 예측모형과 더불어 외환시장의 시장심리지수가 환율 예측에 도움이 되는지 점검해보고자 한다. 그리고 시장심리지수를 사용하여 외환시장 딜러들이 사용하는 반대의견(contrarian opinion) 투자전략에 기반하여 환율을 예측해보았다. 또한 경제여건변수 및 시장심리지수를 종합적으로 활용한 기계학습 모형이 환율 예측력을 높일 수 있는지도 살펴보았다.
본 연구는 크게 네 부분으로 구성되어 있다. 제2장에서는 전통적 환율 예측모형들을 소개하고, 경제여건을 사용한 모형들의 환율 예측력을 검정하였다. 특히 기존 연구에서 많이 분석된 주요 통화(major currency)뿐만 아니라 한국 원화를 포함한 여타 신흥국 통화까지 확장하였다. 선진국 중 캐나다 달러, 스위스 프랑, 영국 파운드화에 대하여 테일러 준칙 모형이 단기적 환율 설명력이 있는 것으로 추정되었으며, 선진국 통화에 비하여 신흥국 통화가 경제여건을 사용한 대다수의 모형에서 장단기 환율 예측력이 높게 나타났다. 기존 연구와 유사하게 시기별, 통화별로 경제변수들의 환율 예측력에 상당한 차이를 보였다.
제3장에서는 시장심리지수와 블룸버그 외환전망의 환율 예측력을 검정하였다. 제2장의 경제여건변수의 환율 예측력 결과와 동일한 여건하에서 비교하기 위하여 월별 데이터로 치환하였으며, 선형 단일 방정식 모형을 적용하였다. 분석 결과, 시장심리지수와 블룸버그 외환전망 모두 유로화에 대하여 단기적으로 환율 예측력이 높게 나타났으나, 다른 주요 통화에 대해서는 확률보행 모형에 비해서 환율 예측력이 떨어지는 것으로 추정되었다.
제4장은 외환시장 딜러들이 사용하는 반대의견 투자전략에 기초하여 환율 예측을 검정하였다. 반대의견 이론에 기초해서 개발된 4개 변수(선물시장 참가자들의 일일심리지수, 현물환율과 최대환율 간의 거리, 과거환율의 수익률, 과거환율의 변동성)들을 사용하여 미래환율을 예측하였으며, 이 중 현물환율과 최대환율 간의 거리가 예측력이 가장 우수한 것으로 나타났다. 또한 환율 예측력은 1주일부터 5년 사이의 모든 환율 예측기간에서 표본 내 적합성, 표본 외 예측력 모두에서 확률보행 모형에 비하여 우수한 것으로 나타났다.
한편 최근에는 머신러닝 알고리즘의 개발이 활성화되고 분석에 이용 가능한 데이터의 양과 질이 개선됨에 따라 머신러닝 알고리즘을 도입하여 시장 분석을 시도하는 많은 연구들이 수행되고 있다. 이에 따라 제5장은 기초여건변수와 시장심리지수를 사용한 머신러닝 모형을 구성하여 환율 변동을 예측하였다. 분석 결과 일반적으로 머신러닝 모형을 기반으로 한 환율 예측이 선형 모형에 비하여 우수한 것으로 나타났으며, 여러 머신러닝 모형 중 합성곱 신경망의 환율 예측력이 높게 나타났다.
제6장에서는 연구 결과를 종합하고 시사점을 제시하였다. 본 연구는 다양한 모형들의 환율 예측력을 점검하나 그 원인을 설명하기는 어려운 한계점을 갖는다. 예를 들어 전통적 환율모형 중 테일러 준칙 모형이 왜 환율 예측력이 높은지에 대한 실증적 해답을 구하기는 어렵다. 또한 신흥국의 경우 선물환 프리미엄 퍼즐(forward premium puzzle)이 나타나지 않는 것(Bansal and Dahlquist 2000)으로 알려져 있는데, 이러한 부분이 환율모형의 예측력과 관련이 있을 것으로 예상되지만, 이를 설명하기 위해서는 시장 투자자, 시장구조 등을 반영한 이론모형에 대한 연구도 향후 필요할 것으로 보인다.
Central bankers, policymakers, and market participants need to predict the future exchange rate movement. However, a well-known puzzle is that exchange rates are difficult to forecast using observable macro fundamental variables. Meese and Rogoff (1983) report that the random walk model is better at predicting exchange rates in out-of-sample forecasts than models reflecting changes in economic fundamentals. A large body of literature has found that, in attempting to solve the Meese‐Rogoff puzzle, the random walk beats fundamentals-based models for periods up to a one‐year forecasting horizon.
This study intends to examine whether the market sentiment index of the foreign exchange market, along with the standardized fundamental-based models, helps predict the exchange rate. Based on the market sentiment index data, we try to predict the exchange rate based on the contrary opinion investment strategy used by foreign exchange market dealers. We also examine whether machine learning models incorporating a wide range of economic data and market sentiment indices can improve exchange rate forecasting.
This study mainly consists of four parts. Chapter 2 re-examines whether fundamental-based models can have prediction power on exchange rates. We examine developing market currencies, including the Korean won, in addition to major currencies. The Taylor-rule model has short-term predictability on the Canadian dollar, Swiss franc, and British pound among major currencies. For most models we analyze, emerging market currencies tend to show higher long-term and short-term predictability than major currencies. However, there is a significant variation in the predictive power of fundamental models over currency and period.
In Chapter 3, the market sentiment index and Bloomberg’s exchange rate forecast are tested in terms of their ability to predict exchange rates. To compare the exchange rate predictability fundamental-based models in Chapter 2, we make them in identical conditions: the data is transformed to monthly, and a single linear equation model is used. Both the market sentiment index and the Bloomberg exchange rate forecast demonstrate high short-term exchange rate predicting power against the Euro. However, they have lower exchange rate forecasting power to other major currencies than the random walk model.
Chapter 4 examines the exchange rate forecast based on the contrast opinion investment strategy used by foreign exchange market dealers. The exchange rate is predicted based on four indicators (daily sentiment index of futures market participants, the distance between the spot exchange rate and the maximum exchange rate, the yield of the past exchange rate, and the volatility of the past exchange rate). Those indicators are made based on the imperfect market theory. Among the indicators, the distance between the exchange rate and the maximum exchange rate has the best predictive power. For forecasting horizons ranging from one week to five years, we find that it outperforms the random-walk model in both in-sample and out-of-sample projections.
As various machine learning algorithms have been developed and the quantity and quality of data accessible for analysis have improved, there have been an increasing number of studies that seek to market analysis based on machine learning algorithms in recent years. Chapter 5 uses fundamental variables and a market sentiment index to predict exchange rate fluctuations using machine learning algorithms. As a result of the analysis, we find that the exchange rate predictions based on the machine learning models are generally superior to the linear model. Among the machine learning models, the convolutional neural network has the best exchange rate forecastability.
Chapter 6 summarizes the research results and suggests implications. Although this study examines the predictive power of exchange rates of various models, it is difficult to explain and identify the cause. Thus, more studies are necessary for discussing the causality. In sum, we test various models for predicting exchange rates and find some empirical results that could beat the random-walk model.
국문요약
제1장 서론
1. 연구의 배경
2. 연구의 의의 및 구성
3. 환율 예측력 평가 방법
제2장 전통적 환율 결정요인과 예측모형
1. 이론적 배경
2. 분석자료
3. 실증분석 결과
4. 동태적 분석
5. 소결
제3장 시장 기대와 환율 예측
1. 이론적 배경
2. 분석자료
3. 실증분석
4. 소결
제4장 환율 예측모형: 반대의견 전략
1. 서론
2. 실증분석 모형
3. 표본 내 적합도 추정 결과
4. 표본 외 예측 추정 결과
5. 강건성 분석
6. 소결
제5장 머신러닝을 활용한 환율 예측
1. 서론
2. 이론적 배경: 시계열 기계학습 모형
3. 실험설계
4. 실증분석 결과
5. 소결
제6장 결론 및 시사점
참고문헌
부록
Executive Summary
판매정보
분량/크기 | 228 |
---|---|
판매가격 | 10000 원 |
같은 주제의 보고서
기본연구보고서
글로벌 인플레이션의 국내파급효과와 경기안정화 정책 분석
2024-12-30
기본연구보고서
대외충격의 자본유출입 효과와 경기안정화 정책 분석
2023-12-29
기본연구보고서
순대외금융자산이 경제안정과 금융 국제화에 미치는 영향 분석
2023-12-29
기본연구보고서
디지털금융을 통한 아프리카 금융포용성 개선 방안 연구
2023-12-30
연구자료
중국의 녹색금융 발전전략과 주요내용
2022-12-30
Policy Analysis
Internationalization of the Korean Won in the Light of the RMB Internationalization
2022-02-25
Working paper
COVID-19 and the Health of Banking Sector in Japan and South Korea: A Comparative Study
2022-07-30
기본연구보고서
국제사회의 부동산 보유세 논의 방향과 거시경제적 영향 분석
2021-12-30
ODA 정책연구
글로벌 ESG 동향 및 국가의 전략적 역할
2021-12-30
연구자료
미국의 스위스 환율조작국 지정 원인 분석 및 평가
2021-11-12
기본연구보고서
신용공급 변동이 경제성장 및 금융위기에 미치는 영향
2020-12-30
기본연구보고서
국내 증권시장에서 외국인 자금 이동 결정요인 분석: 금리와 환율을 중심으로
2020-12-30
기본연구보고서
대외부문 거시건전성 정책 10년의 성과와 개선방안
2020-12-30
기본연구보고서
환율과 경상수지의 구조적 변화와 정책방향
2020-12-30
연구보고서
중국 산업, 얼마나 强한가?: 중국 산업경쟁력의 미시적 토대 분석
2020-02-28
연구보고서
개방경제에서 인구구조 변화가 경상수지 및 대외자산 축적에 미치는 영향분석 및 정책적 시사점
2019-12-30
기본연구보고서
포용적 무역을 위한 중소기업의 국제화 정책방향 연구
2019-12-30
기본연구보고서
내국인 해외증권투자 확대가 외환시장에 미치는 영향
2019-12-30
APEC Study Series
Asia-Pacific Stock Market Connectedness: A Network Approach
2019-10-31
APEC Study Series
Asia-Pacific Stock Market Connectedness: A Network Approach
2019-10-31
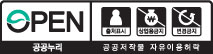
대외경제정책연구원의 본 공공저작물은 "공공누리 제4유형 : 출처표시 + 상업적 금지 + 변경금지” 조건에 따라 이용할 수 있습니다. 저작권정책 참조
콘텐츠 만족도 조사
이 페이지에서 제공하는 정보에 대하여 만족하십니까?