전체보고서
발간물
전체보고서
목록으로
최근 글로벌 인플레이션, 코로나19 팬데믹 등으로 인한 경제 불확실성이 높아지면서 거시경제 전망의 정확성과 속보성에 대한 중요도가 높아지고 있다. 경제 불확실성하에 전망모형의 예측력을 높이기 위해서 잠정적으로 중요한 모든 정보를 활용할 필요가 있다. 이러한 인식과 빅데이터(Big Data)의 등장으로 다양한 데이터를 활용한 경제 전망 연구가 활발히 수행되고 있다. 특히 온라인 검색, 뉴스 및 신문기사 등의 텍스트 데이터는 경제주체들의 심리를 추출하거나 경제 및 금융 상황 모니터링에 활발하게 활용되고 있다. 더불어 빅데이터를 효율적으로 처리하고 분석할 방법론으로 머신러닝이 주목받으면서 거시경제 전망모형으로서 역할이 대두되고 있다. 빅데이터가 전망에 유용하게 활용될 가능성이 높고 새로운 방법론이 개발되면서 빅데이터 기반의 전망과 전통적 거시전망을 종합적으로 분석할 필요가 있다. 이에 본 연구는 빅데이터를 활용하여 경기상황을 대표하는 경제성장률을 단기 전망하고, 전통적 통계모형 및 구조적 거시모형의 전망과 비교하여 예측력이 향상되는지 분석한다. 빅데이터 기반의 전망모형과 구조적 거시모형은 서로 대조되는 특성이 있으므로 각 모형의 결과를 종합적으로 분석하고 향후 경제 전망 연구 방향에 대해 논의한다.
본 연구는 크게 네 부분으로 구성되어 있다. 제2장에서는 빅데이터 기반의 전망에 앞서 벤치마크로 활용할 이론모형인 소규모 개방경제 동태확률일반균형(SOE-DSGE) 모형을 통해 한국의 GDP 성장률을 전망한다. 모형을 베이지안 추정하여 총요소생산성, 정부지출, 통화정책, 해외수요와 해외 통화정책 충격 반응을 분석한 결과, 전반적으로 모형 내 변수들의 외부 충격에 대한 질적인 방향이 현실과 잘 맞는 것으로 나타났다. SOE-DSGE 모형은 방정식 내 구조충격을 명시적으로 고려함으로써 전망뿐만 아니라 경제정책 효과를 분석할 수 있다는 장점이 있다. 다만 모형상의 제약으로 가용할 수 있는 데이터를 모두 활용하지 못한다는 단점이 있다.
제3장에서는 대량의 거시ㆍ금융 지표를 기반으로 머신러닝과 전통적 계량경제 전망모형을 추정하여 미국과 한국의 GDP 성장률 전망치를 구하였다. 머신러닝 기법은 선행연구에서 예측 성과가 좋았던 랜덤포레스트, 엑스지부스트, LSTM과 혼합형 방법론을 사용한다. 전통적 계량경제 전망모형으로는 대량의 예측변수를 활용할 수 있는 동적요인모형(DFM)과 Diffusion Index 모형을 사용하며, 벤치마크로 자기회귀모형(AR)을 함께 살펴본다. 전망 결과 빅데이터를 활용함으로써 GDP 성장률에 대한 예측력이 향상되었으며, 이는 전통적 계량경제 모형보다 머신러닝을 통해 성과를 나타냈다. 미국의 경우 GDP 성장률을 전망하는 머신러닝의 예측력이 계량경제 모형보다 뛰어나며, 1분기 후 RMSE로 측정한 전망 오차가 AR에 비해 최대 34% 줄어드는 것으로 나타났다. 머신러닝의 예측력 향상이 대부분 통계적으로 유의미하지 않지만, 금융위기 기간을 제외한 시기의 1분기 후 전망은 유의미하게 예측력이 뛰어난 것으로 나타났다. 한국은 미국에 비해 머신러닝의 GDP 예측 성과가 뚜렷하지 않지만 2000년대 이후 예측력이 개선되는 모습을 나타낸다. 또한 미국과 달리 머신러닝과 DFM의 예측 성과가 비슷하며, 금융위기 시기의 예측력이 좋은 것으로 나타났다. 따라서 빅데이터를 활용한 머신러닝의 예측력은 국가, 전망시계, 시기, 표본 수에 따라 달라질 수 있음을 유의해야 한다.
제4장에서는 네이버 검색 데이터를 비정형 데이터로 정의하고, 동적모형 평균화 및 선택(DMA 및 DMS)을 이용하여 한국의 GDP 성장률을 전망한다. GDP 성장률 예측변수로 통상적으로 사용되었던 거시ㆍ금융 지표 8개를 기준으로 각 변수와 관련된 네이버 검색어의 검색량을 표준화하여 검색지수를 구축하였다. 온라인 검색 데이터가 특정 시점의 경제성장률 예측에 가장 기여도가 높은 변수를 선정하는 데 유용하다는 아이디어를 기반으로, 동적모형의 선택 확률로 네이버 검색지수를 사용한다. 전망 결과 검색지수를 이용한 DMA 및 DMS가 AR에 비해 예측력이 통계적으로 유의미하게 높으며, 같은 예측변수를 사용한 OLS보다 예측력이 개선되었다. 또한 일반적으로 전망모형들이 평균 회귀적인 모습을 보이는 데 비해 DMA와 DMS는 GDP 성장률의 변곡점들을 잘 예측하는 것으로 나타나 경기변동 시점을 예측하는 데 유용할 것으로 보인다.
제5장에서는 앞에서 구한 한국 전망 결과를 통합하여 비교하고 시사점 및 향후 전망 연구 방향을 제시한다. 첫째, 경제성장률 전망에 빅데이터를 활용함으로써 예측력이 향상되었는데, 이는 더 많은 데이터를 사용하면 잠재적으로 중요한 정보를 추가로 활용할 수 있다는 예상과 부합한다. 둘째, 다양한 소스의 데이터를 발굴하여 거시경제 데이터베이스를 구축할 필요가 있다. 네이버 검색지수를 이용해 구한 전망치는 경제성장률의 급락 및 반등 움직임을 잘 예측하는 반면, 정형 데이터만 사용한 전망치는 급변하는 움직임을 후행 전망하는 것으로 나타났다. 온라인 검색 데이터는 소비자들의 경제심리를 실시간으로 반영하기 때문에 기존의 정형 데이터가 포착하지 못한 정보를 제공하여 급변하는 경기상황을 전망하는 데 유용하게 활용할 수 있다. 마지막으로 데이터베이스 구축과 더불어 경제 전망에 활용할 수 있는 새로운 분석 기법을 개발하고 연구할 필요가 있다. 대량의 예측변수를 사용할 수 있는 통계 방법론이 존재하고 널리 사용되고 있지만, 본 연구에서는 같은 데이터를 사용한 머신러닝의 예측력이 상대적으로 뛰어났다. 머신러닝으로 도출한 경제 전망이 기존의 전망모형 및 경제 전망 전문가의 정성적 판단을 완전히 대체하기는 어렵지만, 최종 전망 또는 경기 상황을 참고할 수 있는 보조지표를 구축하는 데 활용될 수도 있다.
The economic uncertainties arising from recent global inflation and the Covid-19 pandemic have significantly amplified the importance of accuracy and timeliness in macroeconomic forecasts. To enhance the predictive abilities of models, harnessing all potentially relevant information is crucial. The advent of big data has spurred active exploration in economic forecasting research, leveraging additional data dimensions. Notably, text data such as online searches and news articles are widely employed to extract sentiments of economic agents, thereby monitoring economic and financial conditions. Additionally, machine learning has emerged as a pivotal tool in macroeconomic forecasting because it efficiently processes and analyzes big data. Given the potential benefits of big data for forecasting and the ongoing development of new methodologies, a collective analysis of forecasts based on big data and traditional macroeconomic models is essential. In this study, we analyze the predictive ability of short-term GDP growth rate forecasts based on big data against those generated by traditional statistical and structural macroeconomic models. Given the contrasting characteristics between big data-based forecasting models and structural models, we comprehensively analyze the results of each model and discuss implications for future economic forecasting research.
This study largely consists of four parts. In Chapter 2, we utilize a small open economy dynamic stochastic general equilibrium model (SOE-DSGE) to forecast Korea’s GDP growth. This theoretical model serves as a benchmark for comparing against big data-based forecasts. Using a Bayesian framework, the model examines the impacts of various shocks, such as those related to total factor productivity, government spending, monetary policy, foreign demand, and foreign monetary policy. The findings reveal that the response of model variables to external shocks align with real-world outcomes. One of the strengths of the SOE-DSGE model is that it explicitly includes structural shocks, allowing us to analyze not only forecasts but also the effects of economic policies. However, a limitation is its inability to fully leverage available data due to inherent model constraints.
In Chapter 3, we estimate machine learning and traditional econometric models based on a large set of macroeconomic and financial indicators to obtain forecasts of GDP growth in the United States and South Korea. We consider machine learning methods that have shown good predictive performance in previous studies, such as random forests, XGBoost, LSTM, and hybrid methods. For conventional econometric models, we employ the autoregressive model (AR) as a benchmark, along with the Dynamic Factor Model (DFM) and the Diffusion Index Model, both capable of utilizing a large number of predictors. The findings underscore the positive impact of utilizing big data on enhancing the predictive ability of GDP growth forecasts, particularly through machine learning. In the case of the U.S., machine learning outperforms econometric models, reducing forecast errors by up to 34% (measured by RMSE) compared to the AR model at a one-quarter forecast horizon. Although many of these improvements lack statistical significance, machine learning exhibits significant forecasting performance one quarter ahead when excluding periods of financial crises. For South Korea, the performance of machine learning in GDP forecasting is not as pronounced as in the U.S., but improvements have been evident since the 2000s. Unlike in the U.S., both machine learning and the DFM exhibit similar predictive abilities, notably performing well during financial crises periods. Consequently, it’s crucial to note that the forecast performance of machine learning models using big data may vary based on factors such as the country, forecast horizon, time period, and sample size.
In Chapter 4, we classify Naver search data as unstructured and employ dynamic model averaging and selection (DMA and DMS) to predict South Korea’s GDP growth rate. Utilizing eight widely accepted macro-financial indicators as predictors of GDP growth, we create a search index by standardizing the search volume of Naver terms linked to each variable. Based on the idea that online search data is useful for selecting the most influential predictors of economic growth at specific junctures, we integrate the Naver search index as the selection probability of the dynamic model. Our findings reveal that DMA and DMS incorporating the search index, exhibit significantly superior forecasting abilities compared to AR, and also outperforms the OLS employing the same predictors. Moreover, while forecasting models typically exhibit regression towards the mean, DMA and DMS excel in predicting turning points in GDP growth, making them useful for predicting economic fluctuations.
Chapter 5 synthesizes findings from preceding chapters and compares forecasts for Korea, offering insights into future economic forecasting research. Firstly, our results affirm that employing big data enhances the accuracy of economic growth forecasts, which is consistent with the expectation that a richer dataset can unveil important additional information. Secondly, it is necessary to construct a comprehensive macroeconomic database by sourcing information from diverse channels. Our findings show that forecasts integrating the Naver Search Index demonstrate proficiency in predicting sharp fluctuations in economic growth compared to forecasts reliant solely on structured data, indicating the timeliness of online search data in reflecting real-time consumer economic sentiment. This highlights its utility in forecasting evolving economic landscapes, capturing insights beyond existing structured datasets. Lastly, in addition to building databases, we need to innovate and explore new analytical methodologies for economic forecasting. While statistical methods utilizing numerous predictors exists and are widely used, our study reveals the relatively superior predictive performance of machine learning when applied to the same dataset. Although it is difficult to entirely replace existing forecasting models or the qualitative judgment of economists, machine learning-based economic forecasts can serve as supplementary indicators that can be used as a reference for the final forecast or the assessment of the economic landscape.
국문요약
차 례
제1장 서론
1. 연구의 배경과 목적
2. 연구의 내용과 구성
제2장 국제거시경제 전망모형 선행연구
1. 도입
2. 선행연구
3. 모형 경제
4. 모수 추정 및 모형 평가
5. 소결
제3장 빅데이터ㆍ머신러닝 거시경제지표 예측
1. 도입
2. 선행연구 및 전망모형
3. 국내외 거시경제지표 예측
4. 전망모형 간 비교ㆍ평가
5. 시사점 및 소결
제4장 비정형 데이터를 이용한 거시경제 전망모형
1. 도입
2. 전망모형
3. 모형 추정 및 전망 예측
4. 시사점 및 소결
제5장 결론 및 시사점
1. 연구결과 요약
2. 시사점
참고문헌
부록
Executive Summary
판매정보
분량/크기 | 204 |
---|---|
판매가격 | 10,000 원 |
같은 주제의 보고서
기본연구보고서
홍콩의 경제·사회 변화에 대한 평가와 시사점
2024-12-31
연구자료
인도적 지원이 개발도상국 경제성장에 미치는 영향 분석: 2015년 네팔 지진을 중심으로
2024-12-31
APEC Study Series
Economic Factors Affecting Birth Rates in APEC Economies
2024-12-13
APEC Study Series
Economic Factors Affecting Birth Rates in APEC Economies
2024-12-13
중장기통상전략연구
동남아 주요 5개국의 통상전략과 경제성장 경로 : 수출주도성장전략의 평가와 전망
2024-10-11
APEC Study Series
Evolving Agendas in APEC Ministerial Meetings: Tracing the Changing Focus of Economic Cooperation in Asia-Pacific Region
2024-10-02
APEC Study Series
Evolving Agendas in APEC Ministerial Meetings: Tracing the Changing Focus of Economic Cooperation in Asia-Pacific Region
2024-10-02
ODA 정책연구
Assessing Vietnam’s Progress towards Sustainable Development Goals: A Comprehensive Review
2023-12-29
기본연구보고서
대러 경제 제재가 러시아 경제에 미치는 영향과 한-러 경제협력 안정화 방안
2023-12-29
연구보고서
중동부유럽으로의 EU 확대 평가와 향후 전망
2023-12-29
Working paper
Resilience of Faith: Post-Covid Religious Trends and the Effect of Ecclesiastical Policy in the United States
2023-12-29
세계지역전략연구
한-인도 해운·항만산업 협력방안 연구
2023-12-29
기타
만화로 보는 세계경제 2023
2023-12-23
Working paper
Industrial Policy, Rise of Skilled Labor, and Firm Growth in the Early Stage of Economic Development
2023-12-15
Working paper
An Analysis on the Regional Integration of Northeast Asia by Developing NARCI (Northeast Asia Regional Cooperation Index)
2023-10-27
연구자료
국제사회의 성평등 무역규범 도입 현황과 한국의 정책과제
2023-05-06
기본연구보고서
주요국의 탄소중립과 그린성장전략에 관한 연구: EU, 미국, 중국, 일본을 중심으로
2022-12-30
기본연구보고서
코로나19 위기와 기업경쟁구도 변화: 과거 경제위기와의 비교를 중심으로
2022-12-30
연구보고서
포스트 코로나 시대 주요국의 통화·재정정책 방향과 시사점
2021-12-30
세계지역전략연구
카타르의 지속가능한 성장 기반 구축 전략과 협력 시사점
2022-10-31
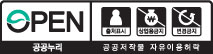
대외경제정책연구원의 본 공공저작물은 "공공누리 제4유형 : 출처표시 + 상업적 금지 + 변경금지” 조건에 따라 이용할 수 있습니다. 저작권정책 참조
콘텐츠 만족도 조사
이 페이지에서 제공하는 정보에 대하여 만족하십니까?