Working Papers
PUBLISH
Working Papers
To list
Developing an International Macroeconomic Forecasting Model Based on Big Data
Economic growth, Economic outlook
Author Yaein Baek, Sang-Ha Yoon, Hyun Hak Kim, and Jiyun Lee Series 23-24 Language Korean Date 2023.12.29
The economic uncertainties arising from recent global inflation and the Covid-19 pandemic have significantly amplified the importance of accuracy and timeliness in macroeconomic forecasts. To enhance the predictive abilities of models, harnessing all potentially relevant information is crucial. The advent of big data has spurred active exploration in economic forecasting research, leveraging additional data dimensions. Notably, text data such as online searches and news articles are widely employed to extract sentiments of economic agents, thereby monitoring economic and financial conditions. Additionally, machine learning has emerged as a pivotal tool in macroeconomic forecasting because it efficiently processes and analyzes big data. Given the potential benefits of big data for forecasting and the ongoing development of new methodologies, a collective analysis of forecasts based on big data and traditional macroeconomic models is essential. In this study, we analyze the predictive ability of short-term GDP growth rate forecasts based on big data against those generated by traditional statistical and structural macroeconomic models. Given the contrasting characteristics between big data-based forecasting models and structural models, we comprehensively analyze the results of each model and discuss implications for future economic forecasting research.
This study largely consists of four parts. In Chapter 2, we utilize a small open economy dynamic stochastic general equilibrium model (SOE-DSGE) to forecast Korea’s GDP growth. This theoretical model serves as a benchmark for comparing against big data-based forecasts. Using a Bayesian framework, the model examines the impacts of various shocks, such as those related to total factor productivity, government spending, monetary policy, foreign demand, and foreign monetary policy. The findings reveal that the response of model variables to external shocks align with real-world outcomes. One of the strengths of the SOE-DSGE model is that it explicitly includes structural shocks, allowing us to analyze not only forecasts but also the effects of economic policies. However, a limitation is its inability to fully leverage available data due to inherent model constraints.
In Chapter 3, we estimate machine learning and traditional econometric models based on a large set of macroeconomic and financial indicators to obtain forecasts of GDP growth in the United States and South Korea. We consider machine learning methods that have shown good predictive performance in previous studies, such as random forests, XGBoost, LSTM, and hybrid methods. For conventional econometric models, we employ the autoregressive model (AR) as a benchmark, along with the Dynamic Factor Model (DFM) and the Diffusion Index Model, both capable of utilizing a large number of predictors. The findings underscore the positive impact of utilizing big data on enhancing the predictive ability of GDP growth forecasts, particularly through machine learning. In the case of the U.S., machine learning outperforms econometric models, reducing forecast errors by up to 34% (measured by RMSE) compared to the AR model at a one-quarter forecast horizon. Although many of these improvements lack statistical significance, machine learning exhibits significant forecasting performance one quarter ahead when excluding periods of financial crises. For South Korea, the performance of machine learning in GDP forecasting is not as pronounced as in the U.S., but improvements have been evident since the 2000s. Unlike in the U.S., both machine learning and the DFM exhibit similar predictive abilities, notably performing well during financial crises periods. Consequently, it’s crucial to note that the forecast performance of machine learning models using big data may vary based on factors such as the country, forecast horizon, time period, and sample size.
In Chapter 4, we classify Naver search data as unstructured and employ dynamic model averaging and selection (DMA and DMS) to predict South Korea’s GDP growth rate. Utilizing eight widely accepted macro-financial indicators as predictors of GDP growth, we create a search index by standardizing the search volume of Naver terms linked to each variable. Based on the idea that online search data is useful for selecting the most influential predictors of economic growth at specific junctures, we integrate the Naver search index as the selection probability of the dynamic model. Our findings reveal that DMA and DMS incorporating the search index, exhibit significantly superior forecasting abilities compared to AR, and also outperforms the OLS employing the same predictors. Moreover, while forecasting models typically exhibit regression towards the mean, DMA and DMS excel in predicting turning points in GDP growth, making them useful for predicting economic fluctuations.
Chapter 5 synthesizes findings from preceding chapters and compares forecasts for Korea, offering insights into future economic forecasting research. Firstly, our results affirm that employing big data enhances the accuracy of economic growth forecasts, which is consistent with the expectation that a richer dataset can unveil important additional information. Secondly, it is necessary to construct a comprehensive macroeconomic database by sourcing information from diverse channels. Our findings show that forecasts integrating the Naver Search Index demonstrate proficiency in predicting sharp fluctuations in economic growth compared to forecasts reliant solely on structured data, indicating the timeliness of online search data in reflecting real-time consumer economic sentiment. This highlights its utility in forecasting evolving economic landscapes, capturing insights beyond existing structured datasets. Lastly, in addition to building databases, we need to innovate and explore new analytical methodologies for economic forecasting. While statistical methods utilizing numerous predictors exists and are widely used, our study reveals the relatively superior predictive performance of machine learning when applied to the same dataset. Although it is difficult to entirely replace existing forecasting models or the qualitative judgment of economists, machine learning-based economic forecasts can serve as supplementary indicators that can be used as a reference for the final forecast or the assessment of the economic landscape.
Sales Info
Quantity/Size | 204 |
---|---|
Sale Price | 10 $ |
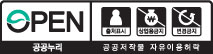
대외경제정책연구원의 본 공공저작물은 "공공누리 제4유형 : 출처표시 + 상업적 금지 + 변경금지” 조건에 따라 이용할 수 있습니다. 저작권정책 참조