Policy Analyses
PUBLISH
Policy Analyses
To list
Exchange Rate Predictability Based on Market Sentiments
Financial policy, Exchange rate
Author Hyosang Kim, Eunjung Kang, Yuri Kim, Seongman Moon, and Huisu Jang Series 21-32 Language Korean Date 2021.12.30
Central bankers, policymakers, and market participants need to predict the future exchange rate movement. However, a well-known puzzle is that exchange rates are difficult to forecast using observable macro fundamental variables. Meese and Rogoff (1983) report that the random walk model is better at predicting exchange rates in out-of-sample forecasts than models reflecting changes in economic fundamentals. A large body of literature has found that, in attempting to solve the Meese‐Rogoff puzzle, the random walk beats fundamentals-based models for periods up to a one‐year forecasting horizon.
This study intends to examine whether the market sentiment index of the foreign exchange market, along with the standardized fundamental-based models, helps predict the exchange rate. Based on the market sentiment index data, we try to predict the exchange rate based on the contrary opinion investment strategy used by foreign exchange market dealers. We also examine whether machine learning models incorporating a wide range of economic data and market sentiment indices can improve exchange rate forecasting.
This study mainly consists of four parts. Chapter 2 re-examines whether fundamental-based models can have prediction power on exchange rates. We examine developing market currencies, including the Korean won, in addition to major currencies. The Taylor-rule model has short-term predictability on the Canadian dollar, Swiss franc, and British pound among major currencies. For most models we analyze, emerging market currencies tend to show higher long-term and short-term predictability than major currencies. However, there is a significant variation in the predictive power of fundamental models over currency and period.
In Chapter 3, the market sentiment index and Bloomberg’s exchange rate forecast are tested in terms of their ability to predict exchange rates. To compare the exchange rate predictability fundamental-based models in Chapter 2, we make them in identical conditions: the data is transformed to monthly, and a single linear equation model is used. Both the market sentiment index and the Bloomberg exchange rate forecast demonstrate high short-term exchange rate predicting power against the Euro. However, they have lower exchange rate forecasting power to other major currencies than the random walk model.
Chapter 4 examines the exchange rate forecast based on the contrast opinion investment strategy used by foreign exchange market dealers. The exchange rate is predicted based on four indicators (daily sentiment index of futures market participants, the distance between the spot exchange rate and the maximum exchange rate, the yield of the past exchange rate, and the volatility of the past exchange rate). Those indicators are made based on the imperfect market theory. Among the indicators, the distance between the exchange rate and the maximum exchange rate has the best predictive power. For forecasting horizons ranging from one week to five years, we find that it outperforms the random-walk model in both in-sample and out-of-sample projections.
As various machine learning algorithms have been developed and the quantity and quality of data accessible for analysis have improved, there have been an increasing number of studies that seek to market analysis based on machine learning algorithms in recent years. Chapter 5 uses fundamental variables and a market sentiment index to predict exchange rate fluctuations using machine learning algorithms. As a result of the analysis, we find that the exchange rate predictions based on the machine learning models are generally superior to the linear model. Among the machine learning models, the convolutional neural network has the best exchange rate forecastability.
Chapter 6 summarizes the research results and suggests implications. Although this study examines the predictive power of exchange rates of various models, it is difficult to explain and identify the cause. Thus, more studies are necessary for discussing the causality. In sum, we test various models for predicting exchange rates and find some empirical results that could beat the random-walk model.
Sales Info
Quantity/Size | 228 |
---|---|
Sale Price | 10 $ |
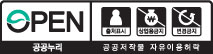
대외경제정책연구원의 본 공공저작물은 "공공누리 제4유형 : 출처표시 + 상업적 금지 + 변경금지” 조건에 따라 이용할 수 있습니다. 저작권정책 참조