PUBLISH
KIEP Opinions
Navigating the AI Revolution
- Author Hongseok Choi
- Series295
- Date2024-08-06
He turned to face the machine. “Is there a God?”
The mighty voice answered without hesitation, without the clicking of a single relay.
“Yes, now there is a God.”
Fredric Brown, 1954, “Answer”
I’m subscribed to three of the major generative AI services, ChatGPT (OpenAI), Claude (Anthropic), and Gemini (Google). When I don’t get what I want with ChatGPT, I switch to Claude. When Claude frustrates me after a good deal of helpful (and impressive) answers, I switch to Gemini. When Gemini does its share, I switch back to ChatGPT. Usually I ultimately get what I want in this cycle, but when I don’t and when I figure out a solution myself, the outcome is satisfactory all the same: I’m not entirely dumber than these AIs yet.
But probably not for long. The speed at which generative AI is progressing is simply astounding. As you may remember, ChatGPT was released in November 2022, only 1 year and 8 months ago. It was initially based on GPT-3.5 (released previously in March 2022) and, via GPT-4 (March 2023), is now using GPT-4o (May 2024). After GPT-3, OpenAI is not disclosing technical details, but GPT-4 is estimated to have 1.76 trillion parameters. Compare this number with GPT-3.5’s 175 billion and GPT-1’s (June 2018) 117 million.
Apparently, model size matters. When ChatGPT was first released, I shrugged off the hype because all it was good at seemed to be apologizing for its wrong, albeit eloquent, answers. Now I think that the chatbots, at least used in tandem, are smarter than many college students (including myself back when I was one). I mean, “GPT-4 beats 90% of lawyers trying to pass the bar.”, Starting September 2023, we can verbally communicate with ChatGPT. When I first tried it recently, for a few seconds I had to wonder if there was another human being on the other side, i.e., ChatGPT would pass the Turing test. So much so scientists are now trying to move the goalposts (“ChatGPT broke the Turing test—the race is on for new ways to assess AI,” Celeste Biever, 25 July 2023, Nature).
To put all this into perspective, let me briefly go over the strand of AI history that led to ChatGPT. ChatGPT, or GPT, is an example of artificial neural networks, or ANNs, which are inspired by the actual, biological neural networks in our head. I am no expert in AI technology, but as far as I understand, an ANN consists of connected nodes (neurons), and training an ANN means properly adjusting the nature (e.g., strength) of the connections. The simple regression model y_i=a+bx_i+ε_i can be interpreted as an ANN: The input node x is connected to the output node y, and their connection is represented by the parameters a and b. Suppose you want an AI that predicts a child’s academic performance (y_i) given his or her parents’ income (x_i). Then you find the values a ̂ and b ̂ of a and b that best fit a given set of data (in an appropriate sense, analytically or numerically); and once the estimation, or training, is done, the AI is ready to output the most likely level of academic performance a ̂+b ̂x_i for a given level of income x_i. Of course, an actual ANN has a lot more nodes and connections and elaborate structures (starting with nonlinearity).
The idea of ANN was first proposed and studied in a 1943 paper titled “A Logical Calculus of the Ideas Immanent in Nervous Activity” by Warren McCulloch and Walter Pitts, and the McCulloch-Pitts neuron, or perceptron, was physically realized in 1958 by Frank Rosenblatt. After the 1950s, interest in ANN, or AI in general, seems to have fluctuated, going through two “AI winters” (the late 1970s and from the late 1980s to early 1990s), until the breakthroughs in the 2000s and 2010s. Certainly, important results continued to be published prior to the 2000s, but even when interest in AI was revived in the 1980s, it was not exactly about ANN but rather about expert systems, an example of which is IBM’s Deep Blue that beat the chess grandmaster Garry Kasparov in 1997. (An expert system consists of manually coded knowledge and rules.)
Then comes Geoffrey Hinton et al.’s (2006) paper, which is widely regarded as having ushered in the age of deep learning by introducing a layerwise pretraining technique. (Deep learning uses “deep” ANNs with many layers of nodes where a layer, well, is a group of nodes.) And in 2017, a mere seven years ago, eight scientists at Google published the paper (Vaswani et al., 2017) that expounded the very architecture of ANN called transformer (the T in GPT) that was to lead to the creation of GPT-1 by OpenAI the next year.
Compare the progress of ANN between 1943 and 2018 with that between 2018 and 2024. Given that, clearly, I’m not even listing all the major chatbots, which are themselves only the tip of the iceberg (think other AIs specializing in images, videos, and speech), the whole path of progress could well be described, at least casually, as exponential. Where would this exponential path lead us to? Recently on June 8, OpenAI CTO Mira Murati said as follows in an interview:
Interviewer: How quickly will [GPT] get to, you know, maybe, human-level intelligence?
Murati: These systems are already human-level in specific tasks, and of course in a lot of tasks, they’re not. If you look at the
trajectory of improvement, systems like GPT-3 were maybe, let’s say, toddler-level intelligence. And then, systems like GPT-4
are more like smart high schooler intelligence. And then, in the next couple of years, we’re looking at PhD-level intelligence
for specific tasks.
By “the next couple of years,” she is alluding to GPT-5. And judging from the fact that she understated the levels of intelligence for GPT-3 and 4, I suspect that GPT-5 may be smarter than PhDs (yes, for specific tasks, i.e., for the very tasks PhDs are better than others at). If a PhD degree is any indication of representative human intelligence, then Murati’s statement must be referring to AGI (artificial general intelligence). In two years.
So AI is already very capable, continues to progress, quickly, and has immeasurable potential. What would be the role of the government in all of this?
1. Invest in AI companies. AI will transform our way of life, it will replace more and more jobs, and eventually most of the value added will come from AI. In anticipation of all these profound changes, the best-case scenario would of course be that Korea houses its own OpenAIs, but AI development is well-known to be subject to economies of scale (computing power, resources for long-term, innovative research, etc.), and if it’s therefore hard to compete with the long list of existing frontier companies (OpenAI alone is valued at $80 billion as of February 2024, and OpenAI CEO Sam Altman is now seeking trillions of dollars to reshape the global semiconductor industry ), it seems necessary for the Korean government to consider strategic investments—substantial stake acquisitions―in these companies to secure the country’s place in the AI-driven future. Indeed, Korea’s public investment funds have already made significant investments in the frontrunners. For example, according to its 13F-HR for the first quarter of this year, KIC’s top six US stock holdings are Microsoft, Apple, Nvidia, Alphabet (Google), Amazon, and Meta (in this order), all of which are playing central roles in the advancement of AI, and KIC’s investments in these companies amount to $9.9 billion, or a quarter of its total US stock investments.
But however much the funds are currently invested in this technology, it isn’t likely enough. Furthermore, there’s no guarantee in the ever-changing AI industry that the current frontrunners will remain at the forefront. Then there are the uncertain market conditions (high debt, geopolitical tensions, etc.) that make any investment decisions now particularly difficult. Therefore, we must allocate more public resources toward better monitoring the development of AI and related market conditions and toward achieving the optimal level of investment in the technology. If necessary, efforts must also be made to convey the necessity, urgency, and difficulty of this task to the public.
2. Subsidize AI development. In fact, what would come first to the economist’s mind when he or she thinks about the role of the government in the development of any (beneficial) technology would be correcting for positive externalities using subsidies. And indeed, the Korean government announced just last month that it had allocated KRW 1.1 trillion (USD 797 million) for AI R&D in the 2025 budget; and back in April, President Yoon Suk Yeol pledged public investment of KRW 9.4 trillion (USD 6.80 billion) in AI and related semiconductors by 2027 and setup of a fund worth KRW 1.4 trillion (USD 1.01 billion) to help innovative AI chipmakers grow. The focus on semiconductor chips is aligned with our comparative advantage in the AI value chain.
In addition to subsidizing innovative chipmakers (i.e., startups), we may also need to consider subsidizing established chipmakers as well just as other major countries and regions—the US, China, the EU and so on—do, on a comparable scale. For example, the US government, in 2022 through the CHIPS and Science Act, allocated $52.7 billion to supporting the semiconductor industry over the next five years, and both of Korea’s top two chipmakers—Samsung Electronics and SK hynix—are planning to make substantial investments in the US, with Samsung Electronics already promised a subsidy and SK hynix waiting for the decision. While the Korean government has also earmarked some of its 2024 budget for promoting foreign investment in cutting-edge industries (the semiconductor industry and others), the amount—KRW 0.2 trillion ($144 million)—pales in comparison to the massive subsidies offered by the US and others. There can be arguments against subsidizing big companies, foreign or domestic, but we can largely mitigate such concerns by following the US example of including profit-sharing provisions.
3. Prepare for the risks. So far I’ve only looked at its bright side, but as is well-known, there are numerous risks associated with AI technology: deepfakes being used in fraud and undermining social trust; privacy and intellectual property right infringements; rationally acting against human interests; and many others. Here, I’ll only mention a couple of points regarding the effects of AI on investment decision making. First of all, we need more research on the properties of AIs as economic decision makers and how their recommendations affect human investors. In other words, we need to subsidize not only the development of AI itself but also the examination of the AI thus developed (which would cost a lot less). People are already using AIs in making investment decisions (a YouTube video titled “ChatGPT Trading Strategy Made 19527% Profit,” posted in March 2023, has 2.9 million views), and there have been many incidents where recommendations by “experts” caused significant losses for retail investors (e.g., the ongoing risk involving Hong Kong-tied ELSs), yet research on this front is only nascent. Also, we must promote AI literacy among the public. AIs can be utilized at all stages of investment decision making in various ways, and how much one can benefit from them depends on his or her proficiency in AI. A widening gap in AI literacy can further exacerbate the existing wealth inequality.
With the importance of public policies duly acknowledged, what’s truly intriguing on a personal level is the questions sci-fi novels and movies pose. The short story quoted at the beginning of this piece continues and ends as follows:
Sudden fear flashed on the face of Dwar Ev. He leaped to grab the switch.
A bolt of lightning from the cloudless sky struck him down and fused the switch shut.
Should ASI (artificial superintelligence) come, we of lesser intelligence won’t be able to understand it. Maybe the machine in the story brought everlasting peace and abundance for human-kind, and it fused the switch shut so that stupid humans wouldn’t turn it off out of irrational fear. Or maybe it enslaved, smote, or did whatever harm to humankind that it saw fit. In any case, there’s no stopping the wheel of the AI revolution now.
Ph.D., Associate Research Fellow, International Finance Team
Department of International Macroeconomics and Finance
-
KIEP Opinions_no295_final.pdf (321.89KB / Download 1,051회)
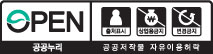
대외경제정책연구원의 본 공공저작물은 "공공누리 제4유형 : 출처표시 + 상업적 금지 + 변경금지” 조건에 따라 이용할 수 있습니다. 저작권정책 참조