Conference Proceedings
Publications
Conference Proceedings
To list
An Analysis of the EvolutaAn Analysis of the Evolution and Factors of the Financial Crisis Using Complex Systems and Machine Learningon and Factors of the Financial Crisis Using Complex Systems and Ma
international finance, financial crisis
Author Young Sik Jeong, Gab Jin Oh, Wontae Han, Yaein Baek, Eunjung Kang, and Yuri Kim Series 22-17 Language Korean Date 2022.12.30
The shadow of the global financial crisis has been looming recently. Prices of almost all assets have plummeted, including stocks, bonds, digital currencies, and real estate, with some vulnerable emerging countries falling into a foreign exchange crisis. In addition, credit supply to the private sector is also slowing, and corporate defaults are increasing. Will this time be different? Or is another financial crisis on the horizon, only in a different form? This is a matter of great concern both on the international and national arenas. Therefore, this study seeks to discover valuable clues from previous cases and the use of new methodologies. We look at network patterns prior to and following the previous financial crises, factors that predict financial crises, and how one financial crisis leads to another financial crisis; and based on this, we diagnose the current situation and identify potential risk factors looking forward.
This study largely consists of five parts, excluding Chapter 1 (Introduction) and Chapter 2 (Existing Research on Financial Crisis: Focusing on Causes and Development Process). In Chapter 3, we examine the relationship between the characteristics of financial crises and the network structure of financial market participants using micro data. During a financial crisis, a high degree of synchronization among heterogeneous economic agents tends to impact the characteristics of the network, resulting in a statistically significant change in the network structure. The connectivity of corporate and bank networks has been found to be very closely related to indicators of financial market risk and volatility. These characteristics were consistently found in domestic and international stock markets, Korea’s social media, and U.S. syndicated loan data. Also, recent network analysis data on the Korean stock market and social media show a strong pattern of synchronization among heterogeneous agents, similar to previous financial crisis periods. In particular, the distribution of correlations among individual companies in the Korean stock market in 2022 is similar to that during the 1992 Nordic financial crisis and the 2011 European fiscal crisis, and is approaching the distribution shown during the 2008 global financial crisis. This indicates the recent increase in risks within the Korean financial market.
In Chapter 4, this study analyzes cross-border capital flows through network methodologies from the macro-perspective. The analysis focuses on the 2008 global financial crisis and 2020 pandemic crisis by deploying IMF CPIS and BIS LBS data. Based on the IMF CPIS dataset, we find that the slump in international capital flows was stronger during the 2008 crisis compared to the 2020 pandemic, and its contraction was more persistent during the 2008 crisis. International capital flows sunk abruptly from the start of the 2020 pandemic crisis, but bounced back quickly after the second half of 2020. One rationale for this observation could be the different characteristics of the crisis triggers involved: i.e, the intrinsic defects of the financial system exposed through the U.S. subprime mortgages served as the trigger in 2008, whereas the trigger for the 2020 pandemic was an exogenous outbreak of infectious diseases. Examining banking flows from BIS LBS, we find Japan and Germany were the main fund suppliers during 2008 and 2020 crises while the demand for banking funds during these crises mainly originated from the U.S. and UK. This pattern tends to be in the opposite during normal phases. We can gather from the BIS LBS data that the network connectivity was more damaged after the onset of the 2008 crisis, but the contraction of banking flows was more severe during the 2020 crisis. HHI (Herfindahl-Hirschman Index) for banking flows suggests that the financial network became concentrated on a few lending and borrowing countries and this escalated risk during the 2008 crisis, but the same pattern was not found in 2020. We must leave diagnosis on cross-border capital flows in 2022 for future study due to the lack of recent data in CPIS and LBS.
In Chapter 5, we use machine learning techniques to examine which factors are important in predicting financial crises. For the entire period (1870-2017), our random forest model shows that the top six most important predictors are the slope of the yield curve, CPI, consumption, debt service ratio, equity return and public debt. According to our analysis, the recent developments of these six predictors indicate an increase in the possibility of a financial crisis. Recently, major countries are showing inverted yield curves and the CPI recorded its largest increase in 40 years since the 1980s. In addition, the slowdown in consumption, increase in the debt service ratio, plunge in stock prices, and high government debt are also factors that indicate the possibility of a financial crisis. In the case of the global financial crisis (2007- 2008), the main factors are the yield curve slope, debt service ratio, consumption, CPI, public debt and equity return, in order of contribution to prediction. Compared to the entire period, the rankings of the debt service ratio and public debt have risen. In predicting the Nordic banking crisis (1988-1993), the yield curve slope, CPI, debt service ratio, consumption, equity return and public debt are important and the contribution of the debt service ratio increased. Therefore, the recent global inflation suggests that the future financial crisis may be closer to the Nordic crisis than the global financial crisis.
In Chapter 6, as a result of examining various past financial crisis case groups through system dynamics (causal loop diagram), five characteristics were found in common, although the appearance of financial crises was different for each case group. The first was a feedback loop that strengthens credit expansion. A monetary easing policy and various factors combine to expand credit, and as this is also combined with achievements such as high growth, rising asset prices, high profits at financial institutions, and stable currency values, a feedback loop structure emerges in which credit continues to expand. Next, a feedback loop that strengthens credit expansion led to the accumulation of financial crisis risk. Specifically, at the national level, high inflation, fiscal account deficit, current account deficit, overvaluation of currency, and expansion of external debt occurred. At the financial institution level, maturity and currency mismatches, increased investment in high-risk assets, and increased loans to low-credit groups appeared. Thirdly, there was the shock that precipitated the financial crisis. Although tight monetary policy is the main factor, various policy and institutional changes, major political and economic events, and changes in investment behavior also act as shock factors. Fourth, there was a risk spreading factor. The degree of spread for each financial crisis depended on the level of risk spread factors (connectivity of networks and synchronization of actions). In addition, the degree of spread of the financial crisis differed depending on whether or not a financial crisis had occurred in a global money-supplying country. Lastly, individual financial crises do not end with themselves, but have the common feature of becoming the seeds for a new crisis. Previous cases show how a new crisis was conceived in the process of responding to a financial crisis and its effects, such as changes in monetary policy, asset prices, fund management behavior, or in the economic structure. When evaluating the recent situation based on these five common characteristics, it was found that the risk of a financial crisis is rising. This is because shocks such as monetary tightening in major countries and escalating geopolitical risks, which can trigger a financial crisis, are adding to the accumulation of risks such as overheating of various asset markets, pursuit of leverage- based high returns, and deterioration of current account balance and external debt of resource-importing countries. Due to structural changes that have occurred since 2008, such as deglobalization, low growth in the Chinese economy, high inflation, a noticeable increase in the size of non-banking and capital markets, and digital asset bubbles, it is likely that any future financial crisis will develop differently from those of the past. In particular, with regard to the financial system, the risk of a financial crisis resulting from market risk rather than credit risk has increased. Furthermore, in terms of cross-country capital flows, the risk of a financial crisis through portfolio investment is now greater than in the past.
Chapter 7 presents the conclusions and policy implications of this study. Two main conclusions were drawn from the study. First, financial crisis is a systemic problem rather than an individual risk factor. Second, when diagnosing the recent situation, the results indicate a risk of a financial crisis spreading beyond some vulnerable emerging countries. These findings yield the following three policy implications. First of all, since the nature of a financial crisis lies in system collapse, policy authorities must strengthen their systemic approach. This is because it is difficult to effectively manage all processes of crisis management, such as risk diagnosis, prevention, shock mitigation, and follow-up management, without a systemic approach. Next, as the risk of a financial crisis has been increasing recently, it is necessary to identify vulnerable factors at the system level and seek ways to mitigate them. The asset bubble in areas such as stocks, bonds, real estate, or digital currencies, together with high-leverage derivative financial products traded under the sustained period of ultra-low interest rates, high volatility of natural resource prices, and portfolio investment channels are being identified as the representative factors of vulnerability. Lastly, combining complex system (network, system dynamics) and machine learning methodology with traditional statistical techniques presents great potential for improving the financial stability in Korea.
Sales Info
Quantity/Size | 293 |
---|---|
Sale Price | 10 $ |
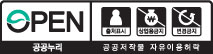
대외경제정책연구원의 본 공공저작물은 "공공누리 제4유형 : 출처표시 + 상업적 금지 + 변경금지” 조건에 따라 이용할 수 있습니다. 저작권정책 참조